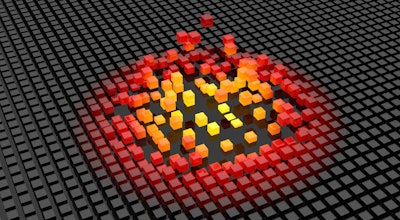
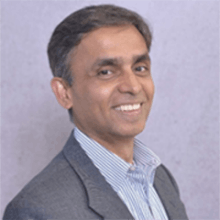
When the average employee or consumer reflects on the impact a supply chain disruption might have on a given company, they are likely to conjure one of the more dramatic and rare scenarios: a tsunami wipes out a crucial manufacturing plant, say, or rising political animosity between two countries leads to closed borders along an integral route.
In my two decades of analytics consulting for companies across multiple industries, I have found that it’s actually the less severe, less headline-grabbing disruptions that really cause much bigger—and more frequent—headaches for supply chain managers. The last-minute variations in customer orders; the sudden changes in a machine’s output; unexpected delays in delivery: These are the minor but impactful supply-chain hiccups that can cumulatively land a major blow to the bottom line.
A thorough understanding of how to leverage data analytics to address supply chain disruptions and strengthen overall processes can help to soften that blow considerably. I can attest that we are already seeing a separation between the leaders and the laggards in this arena, and the leaders are the forward-looking companies who have developed an appetite for data generation, capture and consumption, and even more importantly, have developed an ability to apply sophisticated analytics to that data to gain actionable insights. Other companies, unfortunately, neither have the wherewithal to do this nor have adequately prioritized data-driven decision making and, as time goes on, this will only be more and more to their detriment.
What data steps should companies take first when they determine that it’s time to follow the example of the supply-chain winners? I recommend starting by moving past the vagueness of the “big data” buzzwords and developing a thorough understanding of the three types of data analytics that can be used to address supply chain disruptions. Keep in mind that these three types of data analysis are more like nesting dolls than a menu of selections; your supply-chain team needs to unpack one type before moving on to reap the benefits of the next.
1. Descriptive Analytics
Put simply, descriptive analytics leverage data for the purpose of determining what happened in the past. This method of analysis involves choosing data and running reports designed to generate insights based strictly on what has happened so far to give yourself and your company a clear, useful representation of the past.
In some ways, descriptive analytics may seem the simplest of the three listed here, but it’s also fundamental to the full-scope analytics process. For example, if your colleagues running the supply chain are unaware of historical anomalous patterns in your demand, how can your team begin to plan for the coming year? Your descriptive analysis should be in-depth and reflect every piece of the supply chain process. You may need to run some diagnostics to understand why a disruption happened, especially if it wasn’t something that is easily explainable. Being able to handle supply chain disruptions requires you to understand that they have even happened and to be aware of what was or wasn’t done about it.
2. Predictive Analytics
Predictive analytics allow supply-chain managers to wield the vast quantities of data in their midst—and the insights yielded by descriptive and diagnostic analytics—to make projections about the future. It’s in generating dynamic forecasts that data analytics begins to demonstrate its power.
The strength of predictive analytics isn’t rooted in any sort of magical ability to pinpoint potential black swan events that could disrupt operations. Its power, rather, lies in its ability to forecast events that are much more likely to upheave your supply chain plans, conflicts like weather patterns that may have a profound impact on seasonal products, or consumer trends that are subtle yet have a profound impact on your sales, or which of your machines may start wavering throughout hourly production.
For a number of supply-chain scenarios, an especially useful sub-category of predictive analytics is probabilistic forecasting, which offers a prediction not as a hard number (or “point number” as it’s called in industry) but as a distribution, or a range to plan within. So instead of planning to produce 1,250 units this week to meet customer demand, an analysis might recommend you plan to produce between 825 and 1,675—thereby building more flexibility into your operations.
3. Prescriptive Analytics
Prescriptive analytics builds on smart predictions and incorporates a set of rules, constraints, and operational limitations with a specified corporate goal. Its focus is not only on telling you that a disruption is looming but seeks to help you answer what you should do about it. As useful as this type of analysis can be, it’s also the most difficult, and therefore the least attempted—among these three types. For example, if predictive analytics detect latent trends that manifest themselves in a demand projection that is significantly different from original plans, prescriptive analytics can help mitigate that impact by recommending alternate supply scenarios or by prescribing actions that can help shape the demand in the desired direction.
Prescriptive analytics may represent the pinnacle of the ways in which data analytics can benefit your supply chain and smooth out disruptions, but it’s important not to try to start there. Look for patterns, try to explain them, determine the impact on the present and future, and formulate the moves you will take to make the most of that impact. Taken together, this is what data analytics lets you do.
Suresh Acharya is Professor of Practice at the Robert H. Smith School of Business at the University of Maryland. Suresh has spent over 20 years designing and building statistical and optimization solutions in the areas of Supply Chain Management, Retail Planning, Airline Operations, Logistics, and Pricing and Revenue Management. As a practicing analytical professional and Chief Scientist of JDA Software, Suresh continues to work with Fortune 500 companies in delivering practical algorithmic solutions that demonstrate measurable customer value.